हमारा सुझाव है कि अपने ऐप्लिकेशन से सीधे तौर पर Gemini API और Gemini फ़ैमिली के मॉडल ऐक्सेस करने के लिए, Android के लिए Firebase SDK टूल में Vertex AI का इस्तेमाल करें. यह SDK टूल, Firebase प्लैटफ़ॉर्म का हिस्सा है. इसकी मदद से, फ़ुल-स्टैक ऐप्लिकेशन बनाए और चलाए जा सकते हैं.
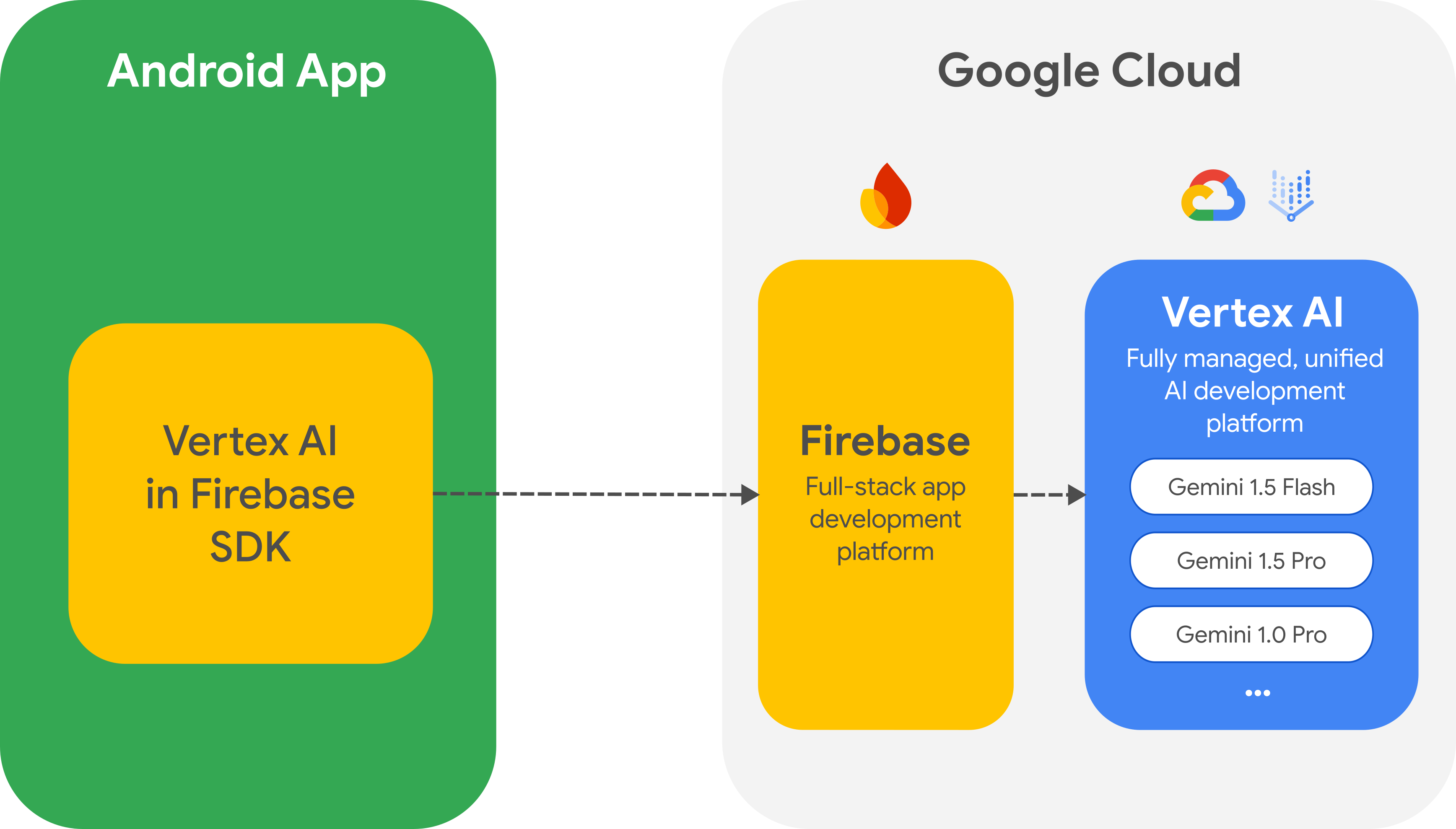
Google के एआई क्लाइंट SDK टूल से माइग्रेट करना
Firebase SDK टूल में Vertex AI, Google AI क्लाइंट SDK टूल जैसा ही है. हालांकि, Firebase SDK टूल में Vertex AI, प्रोडक्शन के इस्तेमाल के उदाहरणों के लिए सुरक्षा के अहम विकल्प और अन्य सुविधाएं उपलब्ध कराता है. उदाहरण के लिए, Firebase में Vertex AI का इस्तेमाल करते समय, इनका भी इस्तेमाल किया जा सकता है:
Firebase App Check, Gemini API को बिना अनुमति वाले क्लाइंट के गलत इस्तेमाल से बचाने के लिए.
Firebase रिमोट कॉन्फ़िगरेशन, जिसकी मदद से क्लाउड में अपने ऐप्लिकेशन के लिए वैल्यू को डाइनैमिक तौर पर सेट और बदला जा सकता है. उदाहरण के लिए, मॉडल के नाम. इसके लिए, आपको अपने ऐप्लिकेशन का नया वर्शन रिलीज़ करने की ज़रूरत नहीं है.
Firebase के लिए Cloud Storage, ताकि Gemini API के लिए किए गए अनुरोध में बड़ी मीडिया फ़ाइलें शामिल की जा सकें.
अगर आपने अपने ऐप्लिकेशन में Google एआई क्लाइंट SDK टूल को पहले ही इंटिग्रेट कर लिया है, तो आपके पास Firebase में Vertex AI पर माइग्रेट करने का विकल्प है.
शुरू करना
अपने ऐप्लिकेशन से सीधे Gemini API के साथ इंटरैक्ट करने से पहले, आपको कुछ काम करने होंगे. इनमें, प्रॉम्प्ट के बारे में जानना और SDK टूल का इस्तेमाल करने के लिए, Firebase और अपने ऐप्लिकेशन को सेट अप करना शामिल है.
प्रॉम्प्ट आज़माना
Vertex AI Studio में प्रॉम्प्ट आज़माए जा सकते हैं. Vertex AI Studio, प्रॉम्प्ट डिज़ाइन और प्रोटोटाइप बनाने के लिए एक आईडीई है. इसकी मदद से, टेक्स्ट और इमेज के साथ प्रॉम्प्ट की जांच करने के लिए फ़ाइलें अपलोड की जा सकती हैं. साथ ही, किसी प्रॉम्प्ट को सेव करके बाद में उस पर फिर से जाया जा सकता है.
अपने इस्तेमाल के उदाहरण के लिए सही प्रॉम्प्ट बनाना, विज्ञान से ज़्यादा कला है. इसलिए, प्रयोग करना ज़रूरी है. Firebase दस्तावेज़ में जाकर, प्रॉम्प्ट के बारे में ज़्यादा जानें.
Firebase प्रोजेक्ट सेट अप करना और अपने ऐप्लिकेशन को Firebase से कनेक्ट करना
अपने ऐप्लिकेशन से Gemini API को कॉल करने के लिए तैयार होने के बाद, अपने ऐप्लिकेशन में Firebase और SDK टूल सेट अप करने के लिए, Firebase में Vertex AI का इस्तेमाल शुरू करने से जुड़ी गाइड में दिए गए निर्देशों का पालन करें. इस गाइड में दिए गए निर्देशों की मदद से, इस गाइड में बताए गए सभी टास्क पूरे किए जा सकते हैं.
नया या मौजूदा Firebase प्रोजेक्ट सेट अप करें. इसमें, इस्तेमाल के हिसाब से पैसे चुकाने वाले Blaze के प्लान का इस्तेमाल करना और ज़रूरी एपीआई चालू करना शामिल है.
अपने ऐप्लिकेशन को Firebase से कनेक्ट करें. इसमें, अपने ऐप्लिकेशन को रजिस्टर करना और अपने ऐप्लिकेशन में Firebase कॉन्फ़िगरेशन फ़ाइल (
google-services.json
) जोड़ना शामिल है.
Gradle डिपेंडेंसी जोड़ना
अपने ऐप्लिकेशन मॉड्यूल में, यह Gradle डिपेंडेंसी जोड़ें:
Kotlin
dependencies { ... implementation("com.google.firebase:firebase-vertexai:16.0.2") }
Java
dependencies { [...] implementation("com.google.firebase:firebase-vertexai:16.0.2") // Required to use `ListenableFuture` from Guava Android for one-shot generation implementation("com.google.guava:guava:31.0.1-android") // Required to use `Publisher` from Reactive Streams for streaming operations implementation("org.reactivestreams:reactive-streams:1.0.4") }
Vertex AI सेवा और जनरेटिव मॉडल को शुरू करना
GenerativeModel
को इंस्टैंशिएट करके और मॉडल का नाम बताकर शुरुआत करें:
Kotlin
val generativeModel = Firebase.vertexAI.generativeModel("gemini-1.5-flash")
Java
GenerativeModel gm = FirebaseVertexAI.getInstance().generativeModel("gemini-1.5-flash");
Firebase के दस्तावेज़ में, Firebase में Vertex AI के साथ इस्तेमाल करने के लिए, उपलब्ध मॉडल के बारे में ज़्यादा जानें. मॉडल पैरामीटर कॉन्फ़िगर करने के बारे में भी जानें.
अपने ऐप्लिकेशन से Gemini API के साथ इंटरैक्ट करना
SDK टूल का इस्तेमाल करने के लिए, Firebase और अपने ऐप्लिकेशन को सेट अप करने के बाद, अब आपके पास अपने ऐप्लिकेशन से Gemini API के साथ इंटरैक्ट करने का विकल्प है.
टेक्स्ट जनरेट करना
टेक्स्ट जवाब जनरेट करने के लिए, अपने प्रॉम्प्ट के साथ generateContent()
को कॉल करें.
Kotlin
// Note: `generateContent()` is a `suspend` function, which integrates well // with existing Kotlin code. scope.launch { val response = model.generateContent("Write a story about the green robot") }
Java
// In Java, create a `GenerativeModelFutures` from the `GenerativeModel`. // Note that `generateContent()` returns a `ListenableFuture`. Learn more: // https://developer.android.com/develop/background-work/background-tasks/asynchronous/listenablefuture GenerativeModelFutures model = GenerativeModelFutures.from(gm); Content prompt = new Content.Builder() .addText("Write a story about a green robot.") .build(); ListenableFuture<GenerateContentResponse> response = model.generateContent(prompt); Futures.addCallback(response, new FutureCallback<GenerateContentResponse>() { @Override public void onSuccess(GenerateContentResponse result) { String resultText = result.getText(); } @Override public void onFailure(Throwable t) { t.printStackTrace(); } }, executor);
इमेज और दूसरे मीडिया से टेक्स्ट जनरेट करना
ऐसे प्रॉम्प्ट से भी टेक्स्ट जनरेट किया जा सकता है जिसमें टेक्स्ट के साथ-साथ इमेज या अन्य मीडिया भी शामिल हो. generateContent()
को कॉल करते समय, मीडिया को इनलाइन डेटा के तौर पर पास किया जा सकता है (जैसा कि नीचे दिए गए उदाहरण में दिखाया गया है). इसके अलावा, Firebase के यूआरएल के लिए Cloud Storage का इस्तेमाल करके, अनुरोध में बड़ी मीडिया फ़ाइलें शामिल की जा सकती हैं.
Kotlin
scope.launch { val response = model.generateContent( content { image(bitmap) text("what is the object in the picture?") } ) }
Java
GenerativeModelFutures model = GenerativeModelFutures.from(gm); Bitmap bitmap = BitmapFactory.decodeResource(getResources(), R.drawable.sparky); Content prompt = new Content.Builder() .addImage(bitmap) .addText("What developer tool is this mascot from?") .build(); ListenableFuture<GenerateContentResponse> response = model.generateContent(prompt); Futures.addCallback(response, new FutureCallback<GenerateContentResponse>() { @Override public void onSuccess(GenerateContentResponse result) { String resultText = result.getText(); } @Override public void onFailure(Throwable t) { t.printStackTrace(); } }, executor);
कई बार की गई चैट
आपके पास एक से ज़्यादा बार बातचीत करने की सुविधा भी है. startChat()
फ़ंक्शन की मदद से चैट शुरू करें. मैसेज का इतिहास भी दिया जा सकता है. हालांकि, ऐसा करना ज़रूरी नहीं है. इसके बाद, चैट मैसेज भेजने के लिए sendMessage()
फ़ंक्शन को कॉल करें.
Kotlin
val chat = generativeModel.startChat( history = listOf( content(role = "user") { text("Hello, I have 2 dogs in my house.") }, content(role = "model") { text("Great to meet you. What would you like to know?") } ) ) scope.launch { val response = chat.sendMessage("How many paws are in my house?") }
Java
// (Optional) create message history Content.Builder userContentBuilder = new Content.Builder(); userContentBuilder.setRole("user"); userContentBuilder.addText("Hello, I have 2 dogs in my house."); Content userContent = userContentBuilder.build(); Content.Builder modelContentBuilder = new Content.Builder(); modelContentBuilder.setRole("model"); modelContentBuilder.addText("Great to meet you. What would you like to know?"); Content modelContent = userContentBuilder.build(); List<Content> history = Arrays.asList(userContent, modelContent); // Initialize the chat ChatFutures chat = model.startChat(history); // Create a new user message Content.Builder messageBuilder = new Content.Builder(); messageBuilder.setRole("user"); messageBuilder.addText("How many paws are in my house?"); Content message = messageBuilder.build(); Publisher<GenerateContentResponse> streamingResponse = chat.sendMessageStream(message); StringBuilder outputContent = new StringBuilder(); streamingResponse.subscribe(new Subscriber<GenerateContentResponse>() { @Override public void onNext(GenerateContentResponse generateContentResponse) { String chunk = generateContentResponse.getText(); outputContent.append(chunk); } @Override public void onComplete() { // ... } @Override public void onError(Throwable t) { t.printStackTrace(); } @Override public void onSubscribe(Subscription s) { s.request(Long.MAX_VALUE); } });
जवाब स्ट्रीम करना
मॉडल जनरेशन के पूरे नतीजे का इंतज़ार किए बिना, तेज़ी से इंटरैक्शन किया जा सकता है. इसके बजाय, कुछ नतीजों को हैंडल करने के लिए स्ट्रीमिंग का इस्तेमाल करें. जवाब स्ट्रीम करने के लिए,
generateContentStream()
का इस्तेमाल करें.
Kotlin
scope.launch { var outputContent = "" generativeModel.generateContentStream(inputContent) .collect { response -> outputContent += response.text } }
Java
// Note that in Java the method `generateContentStream()` returns a // Publisher from the Reactive Streams library. // https://www.reactive-streams.org/ GenerativeModelFutures model = GenerativeModelFutures.from(gm); // Provide a prompt that contains text Content prompt = new Content.Builder() .addText("Write a story about a green robot.") .build(); Publisher<GenerateContentResponse> streamingResponse = model.generateContentStream(prompt); StringBuilder outputContent = new StringBuilder(); streamingResponse.subscribe(new Subscriber<GenerateContentResponse>() { @Override public void onNext(GenerateContentResponse generateContentResponse) { String chunk = generateContentResponse.getText(); outputContent.append(chunk); } @Override public void onComplete() { // ... } @Override public void onError(Throwable t) { t.printStackTrace(); } @Override public void onSubscribe(Subscription s) { s.request(Long.MAX_VALUE); } });
अगले चरण
- GitHub पर, Firebase में Vertex AI के सैंपल ऐप्लिकेशन की समीक्षा करें.
- प्रोडक्शन के लिए तैयारी करने के बारे में सोचना शुरू करें. इसमें, Gemini API को बिना अनुमति वाले क्लाइंट के गलत इस्तेमाल से बचाने के लिए, Firebase App Check को सेट अप करना भी शामिल है.
- Firebase दस्तावेज़ में, Firebase में Vertex AI के बारे में ज़्यादा जानें.